Demystifying Generative Systems

Demystifying Generative Systems
This is the third part in a series on building a framework for appreciating generative art. In Part I, “Demystifying Generative Art,” Peter Bauman (Monk Antony) builds the case for such a framework. In Part II, “Demystifying Generative Aesthetics,” Bauman examines outputs—the results of generative systems, while he looks at autonomy in Part IV, "Demystifying Generative Autonomy."
Now in Part III, he investigates another layer of the Framework, systems themselves. He spoke to Frieder Nake, Casey Reas, Emily Xie, DEAFBEEF, Matt DesLauriers, Jen Lowe and Patricio González Vivo for the piece.
“Systems aren’t sexy, they hurt to think about for too long, they can hide sloppy thinking behind the mask of complexity, and if they’re working they should be invisible.
But they also rule our lives inside and out so we better get used to putting our attention on them. Maybe art can help build some mental muscles and taste for systems.”
-Ian Cheng
Can you understand a work of generative art just by looking at it? Why use systems to make art in the first place? How do we even go about appreciating systems? What can generative systems achieve that other forms of expression cannot? If you’re reading this and thinking, “I respond to art on a sensory-emotional level only. I don’t need this.” That’s perfectly acceptable but this article is for viewers who wish to supplement that sensory level by seeking the clues artists leave that reveal so much more about their work. By studying systems, we don’t diminish our emotional response; we amplify it through justification or mature it through reconsideration.
A reason the act of viewing alone may not be sufficient to fully engage with systems-based work—including generative art—is that its practitioners themselves have long explained their work in terms of process rather than aesthetics. This has made the understanding of systems central to digital art’s very beginnings. Frieder Nake, one of digital art’s progenitors, told me about his regard for systems:
“The algorithm is a total artifact. This becomes your work. This is the major creative act.” He continued, “It's a fantastic, distant relation between this kind of art and the person who is doing it.” I then asked the icon to explain his thoughts on what he terms “algorithmic art.”
“If you do something that results in visuals and is influenced by randomness, by algorithms and by generating—with the human having set up only the conditions for what is happening—this is algorithmic art.” For Nake, the artist establishes the conditions and those conditions are the art. Hearing Nake speak, it is systems—their nature, construction and his dialogue with them—that inspire his creativity.
Systems, regardless of any single method or process, are a way of thinking about art. Understanding them can bring us closer to the full articulation of an artist’s ideas, which can serve to extend our sensory-critical, phenomenological understanding of the work. For some, this sensory perspective is enough. But it may not be complete—not with generative art. For other collectors and appreciators fanatical enough to dig deeper, they must consider systems.
1. Why Use Systems in Art?
Systems, often machines like computers, appear to some as unnatural—even unhuman—yet the earliest known creative expression by humans is system-based. The Blombos Cave drawings in South Africa demonstrate our ancient, systems-based thinking as the work displays an apparent tiling-based symmetry system. Basket weaving, Islamic tiling art and Mozart’s musical dice game reveal the creative application of systems throughout human history and across civilizations. Systems thinkers also comprise some of the greatest artistic minds of the last one hundred years, including Marcel Duchamp, Anni Albers, Bridget Riley and Nam June Paik, to name a few. In other words, systems thinking has ancient roots and a canonized art-historical legacy.
But why use systems to make art, especially when systems aesthetics can be strikingly similar to traditional methods? This ranges from the hard-edged geometric abstraction of Al Held or Sol LeWitt to the more contemporary mixed media photography of Maria Hassabi, featuring haunting dreamscapes reminiscent of early AI latent-diffusion models.

Then why use systems to make art? Doesn’t it make art harder? System-based artists subvert viewers’ expectations by placing their senses in a subordinate position, compelling them to consider whether perception alone is enough to fully understand. System-based work achieves this radical rethinking by prioritizing process—the production of art through conversation with a method. Picasso’s Guernica is understood as a singular work of art and can be analyzed as such, yet viewing a singular output from a system misleads the viewer; it’s simply incomplete. Matt DesLauriers explained to me: “It's not about the image. One image is just one of thousands or millions of outputs that could come from the system. The system is where the heart of the artwork lies and the heart of my focus.”
So why use systems to make art? What can they achieve that other forms of expression cannot? Systems give artists power. Matt DesLauriers, again, expounded: “That's the one thing that generative art has over a lot of other fields of art, like painting, where you're creating a single static output. With generative art, you're creating a system that can produce many outputs that can be realized in many different media.” Those media include poetry on paper, drawing robots that paint, infinitely animated code and endless more. DesLauriers continues, “Taking a system that can be reconfigured, changed, evolved or iterated upon with different parameters, outputs or iterations is so flexible.”
Understanding systems thus gives the viewer insight into artists’ raison d'être and the most fundamental creative choices they make. Ultimately, exploring a system’s subtlety, originality and logic will unlock a deeper and fuller appreciation of generative work. For digital generative art, that means having, at worst, a basic understanding of common generative systems.
2. Understanding Generative Systems
By understanding the various creative choices that result in a generative system, we more closely approach what the artist shows, receiving a fuller message. We’ll begin with one of the first choices an artist makes: which generative technique to choose.
Understanding Generative Techniques
In the beginning, artists must choose the nature of their system—material, procedural, parametric or AI. Crucially, not all generative methods are digital or code-based.
- Material: A generative system relying on physical elements such as natural forces, manual algorithms or inherent properties of the materials themselves to guide the creation process. Material-generative artists such as Sol LeWitt, Sonya Rapopport and Hans Haacke have work spanning decades and extensive institutional acceptance.
- Procedural: This is likely what most people have in mind when they think of generative systems, as procedures can be computational, like computer algorithms. The core idea is that the artist arranges a system or process that can produce various—typically visual—outputs, often with a degree of randomness or variability, leading to unique and sometimes unpredictable results. William Mapan’s Dragons on fxhash or Erick Calderon’s Chromie Squiggle on Art Blocks serve as examples.
- Parametric: Systems where the artist chooses certain parameters—variables that define system characteristics and behaviors—to control directly, not through chance. This method typically involves co-creation, with artists inviting collectors to participate in the creative process by controlling these special parameter inputs. Examples are Tyler Hobbs and Indigo Mané’s QQL and fxhash’s fx(params).
- Artificial Intelligence (AI): This involves incorporating one of several machine intelligence techniques—including Generative Adversarial Networks (GANs), latent diffusion models (LDMs) and evolutionary algorithms—to generate art work. GANs consist of two neural networks: an image-creating generator and an image-evaluating discriminator. They learn from each other to improve output accuracy, such as in Robbie Barrat’s Nude Portrait series. LDMs like DALL-2 and Midjourney use latent space to reconcile text and image, including the work of Minne Atairu. Finally, evolutionary algorithms use mechanisms inspired by biological evolution—i.e., selection, mutation or crossover—to generate new images. Examples include Yoichiro Kawaguchi and Christa Sommerer.
Understanding Generative Software
Once a technique is chosen, the materials need to be assembled. For digital work, that means choice of software, which profoundly impacts the entire creative process, particularly the options within and outputs of a generative system. There may be a number of reasons for choosing a particular type of software, but common considerations include the feature set, usability, compatibility, performativity, community and accessibility concerns—such as cost.
The case of DEAFBEEF perfectly illustrates how understanding an artist’s software choice can be the key to unlocking their entire oeuvres. He explained to me, “I've chosen my tool set for writing code using C as a programming language because it’s not expected to change. It's less about permanence and more about agency.” Later in our conversation, he made clear the centrality of agency to his entire practice: “Whether it be for coding or making things out of metal, my intent stems from a desire for agency.” For DEAFBEEF and many more artists, the most foundational element of his practice, agency, is linked to his software choice.
Databases such as “That Creative Coding Page’s” software list and Raphaël de Courville’s software guide provide much more technical detail for artists of all kinds—or intrepid collectors—to continue down the software-choice rabbit hole. The important thing is to know how foundational the choice of software is for artists and how many creative decisions it dictates.
Understanding Generative Coding Systems
Now we get to perhaps the core of the entire article, identifying and recognizing the most common generative coding systems that comprise the majority of the digital generative systems you’ll come across. When I spoke to Jen Lowe and Patricio González Vivo, co-authors of The Book of Shaders, about how to gain a richer appreciation for a work’s process, Lowe replied, “There's this particular creative coding pattern language with maybe ten different types of algorithm that people tend to be remixing.”
Let’s examine those then, the visual vocabulary of generative art, which I mostly synthesized from several invaluable sources: Lowe and González Vivo’s The Book of Shaders, Taru Muhonen and Raphaël de Courville’s “That Creative Code Page,” Dan Shiffman’s The Nature of Code and Gene Kogan’s site.
Randomness and Noise
Noise can be a challenge intuitively to grasp but “A Survey of Procedural Noise Functions” describes it as, “the random number generator of computer graphics. It is a random and unstructured pattern.” When completely random, noise can be overwhelming. When adjusted, it can be used to create textures, patterns and effects that make work appear natural and organic. But there are many different types of noise.
- Random noise: Think of glitch art or the white noise of a TV. Truly random noise may not always have sustained visual appeal. Often, artists organize the randomness with a function like Perlin or Simplex noise.
- Perlin noise and flow fields: The motherlode and ubiquitous in digital generative art. With total randomness’s previously described shortcomings, Perlin noise introduces semi-randomness, adding a smoothing organic gradient to random noise. Perlin noise is often used for textures that resemble the natural world—anything from clouds to tree bark to ocean waves. It can be used in many ways but one notable application for generative art is its combination with flow fields.
If any coding term has reached “household-name” status, it’s flow fields, popularized by Tyler Hobbs’s Fidenza but with its own extensive history, as discussed later in Section 3. Shiffman explains flow fields with his unmistakable written flair: “Think of the canvas as a grid. In each cell of the grid lives an arrow pointing in a certain direction—you know, a vector. As a vehicle moves around the canvas, it asks, “Hey, what arrow is beneath me? That’s my desired velocity!” But flow fields have no inherent visual order. Structured randomly, they appear chaotic. With too much order, they can appear boring. Ordered with 2D Perlin noise, they appear sublime.
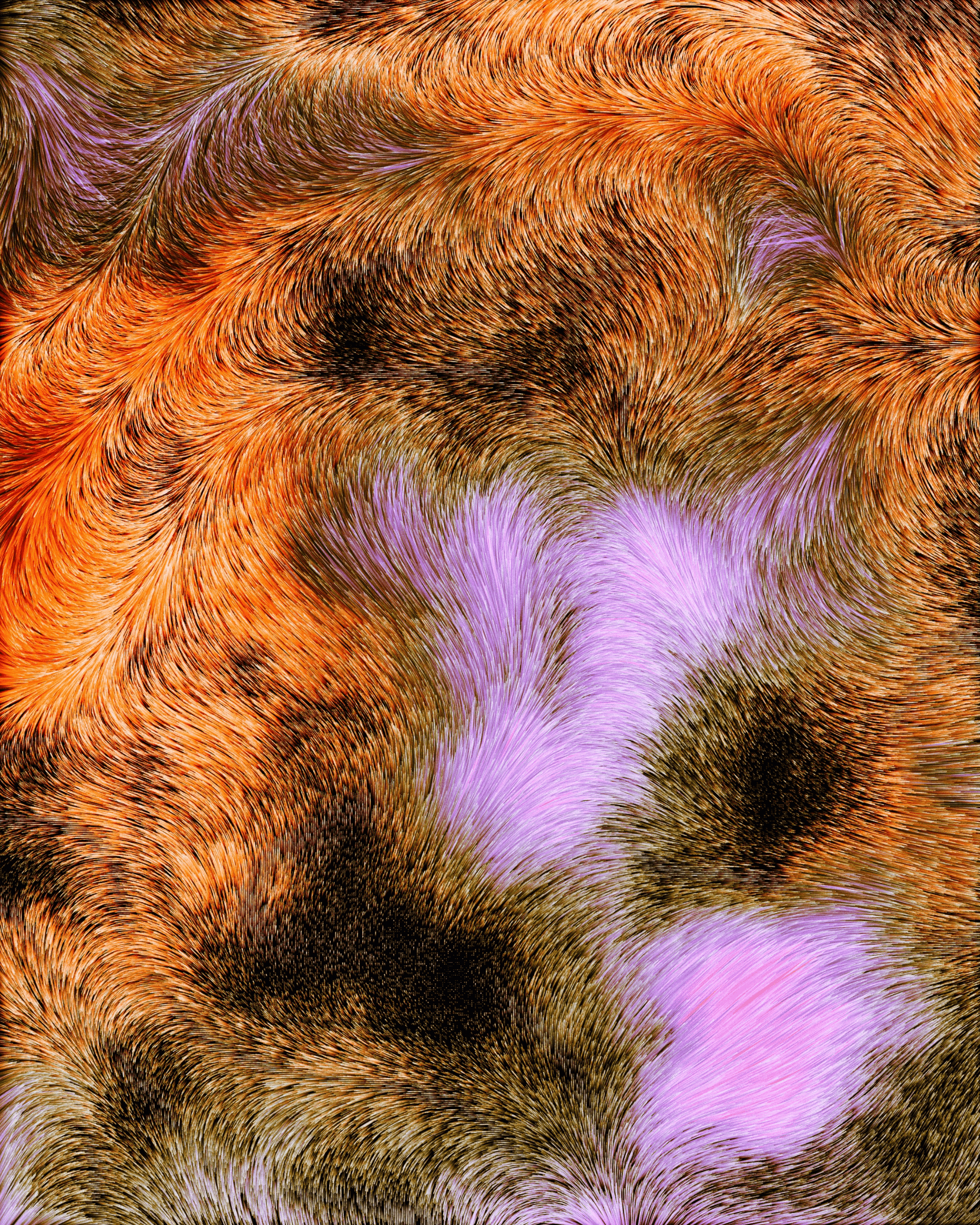
- But there are many other ways to approach flow fields. Flow fields—fundamentally, arranging vectors on a grid—are such an essential element of generative coding that recognizing them is essential. No single artist owns them and work should not be praised or discounted simply for their use.
- Cellular noises: Unlike Perlin noise, which creates smooth gradients, cellular noise produces cell-like structures that can be used to create a variety of effects resembling natural formations such as stone, scales or even organic tissue. The two main types are Voronoi and Worley.
- There are many more noises I didn’t cover but please feel free to dig in.
Signed Distance Functions and Raymarching
A Signed Distance Function (SDF) is a mathematical construct used to describe shapes and surfaces in a continuous space. Artists use them to create and animate detailed 3D scenes while prioritizing efficiency—hence their popularity and innovation stem from the demoscene. An SDF is like a magical ruler for artists, returning the shortest distance from any point in space to the surface of a shape, with the sign indicating whether the point is inside or outside the shape. This concept has become a cornerstone in the field of generative art, especially for creating complex, three-dimensional scenes and animations.
Raymarching’s roots extend to at least computer graphics pioneer Dan Sandin in 1989, with its popularization in the demoscene by Inigo Quilez. This technique uses the spatial information from SDFs to draw shapes in 3D with incredible detail and complexity while maintaining coded elegance.
Fractals
Lines in nature are rarely perfectly straight; neither are shapes of any kind (circles, squares, triangles, etc.). Fractals in computer graphics are used to mimic the organic irregularities of nature. In Benoit Mandelbrot’s The Fractal Geometry of Nature (1975), he mathematically observed how shapes could be split into self-copies like a tree branch. As computers at that time could only make perfect geometric shapes, in 1980 Loren Carpenter brought fractals to computer graphics with his monumental Vol Libre, the first fractal movie. The visual, infinite complexity that fractals achieve makes them fruitful areas to visually explore. The following all make use of a fractal's self-similarity or recursive properties.
- Mandelbrot set: One of the most famous examples of a fractal, a key feature is self-similarity, meaning that if you zoom into the boundary, you will find smaller copies of the entire set, as well as other intricate patterns that resemble the overall shape.
- Cantor set: Another famous fractal pattern, cantor sets introduce recursion—the repeated application of a rule as a feedback loop—to generative patterns. The classic Cantor set is created by starting with a line segment, then repeatedly removing the middle third of each segment. John Maeda explores the tension between simplicity and complexity that fractals afford below.
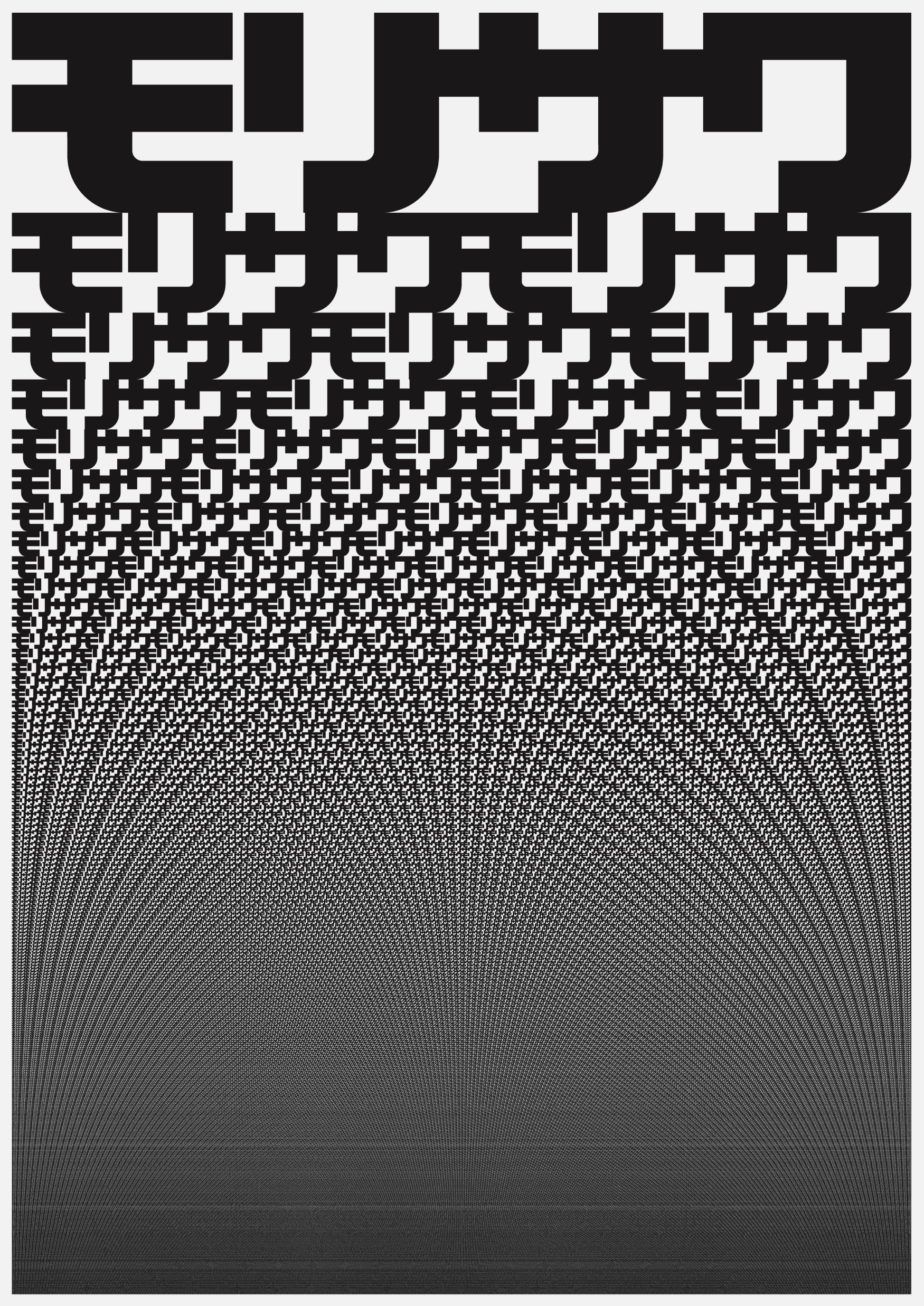
- L-Systems (Tree Fractals): Think Zancan or most tree-based generative art you’ve seen. L-systems, developed in 1968 by Aristid Lindenmayer, model the growth of plants with sophisticated recursive drawing rules.
- Many other fractal types exist to explore, including the Julia set, Sierpiński triangless—recursively subdivided triangles—and the Koch curve.
Patterns
Fractals and noise often involve devising visual meaning from chaotic randomness in the form of recognizable and repeating shapes, otherwise known as patterns. There are countless ways to generate patterns, a fundamental building block of generative coding. Here are some of the most common.
- Space Partitioning/Packing: Shape packing recalls the days of mosaics and Islamic tiling, while space partitioning involves dividing a space into distinct regions according to specific rules. Space partitioning techniques like Voronoi diagrams or Delaunay triangulations create ornate patterns by determining which points are closest to each other. Artists such as Lars Wander have utilized packing methods to produce complex, rule-based work.
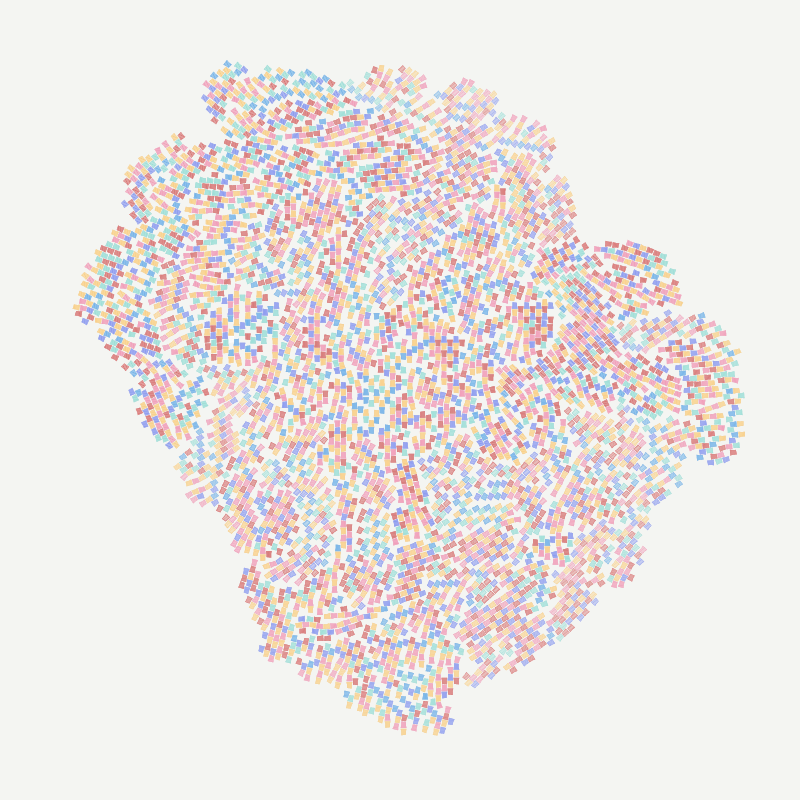
- Dithering: Think of it like pixelization or pixel art. Originally a technique used in printing and digital image processing to create the illusion of color depth with limited palettes, dithering has found its way into generative art. By strategically placing dots of varying sizes and colors, artists can simulate gradients and complex textures. This method is closely related to Georges Seurat's pointillism. In the digital realm, artists such as Andreas Gysin and loackme use dithering techniques like Floyd-Steinberg and Blue Noise to create this century’s pixelated fine art.
- Reaction-Diffusion: These systems model how substances—such as chemicals or fungi—interact and spread to form patterns. The basis for these models originates in the stunning real-world visuals produced by chemical reaction known as the Belousov-Zhabotinsky reaction. This essay by artist ciphrd gives much more background on the popular technique by one of its foremost practitioners, along with Alexander Mordvinstev. These popular, flexible systems enable generative artists to simulate natural phenomena as diverse as animal coat patterns and chemical concentrations.
- Flocking: Systems that simulate the collective behavior of groups, such as birds or fish. Introduced by Craig Reynolds in his seminal 1986 artificial life program, Boids, these algorithms rely on simple rules governing individual agents to produce emergent, complex group dynamics. In generative art, flocking behavior is used to create organic, fluid compositions that capture the essence of movement and interaction. Artist Yoshi Sodeoka is known for his complex use of flocking.
Cellular Automata
Cellular automata (CA) are mathematical models used to simulate complex systems composed of simple, discrete units—cells. CAs, first conceptualized by John von Neumann and Stanislaw Ulam in the 1940s, have become a fundamental tool in generative art. They consist of a grid of cells, each of which can exist in a finite number of states, depending on rules and its neighbors.
The roots of cellular automata lie in the quest to understand self-replicating systems and complex behavior arising from simple rules. John Conway’s "Game of Life," introduced in 1970, brought widespread attention to the potential of CAs. Artists today, such as Manolo Gamboa Naon and Raven Kwok, have continued to pursue the artistic application of CA.
Above are some of the most recognizable building blocks of generative art. With these and many others to choose from, each creative decision by the artist—previously detailed—exposes a hidden conversation that may have otherwise gone unheard. The systems artist speaks to the viewer in the minutiae of their process, revealing so much about their work—their influences, their interests, their very reason for creating in the first place—simply by their creative choices. But we have to listen. And once we’ve heard it, how do we process it all?
3. Appreciating Systems
Categorize
Attempt to narrow down what you know about the system, beginning by following the artist’s creative choices as outlined in Section 2. What does their technique reveal about their artistic vision? How did their choice of software impact each choice along the way, as well as the final outputs? Finally, attempt to decipher or narrow down which coding system they used. How specific can you get? There are many wonderful resources by this space’s greatest thinkers and builders that I’ve linked throughout if you’d like to know more, including resources in Japanese from Kenichi Yoneda and another resource by Gorilla Sun for continuous updates.
But also understand that artists may not always make any of this clear, which is half the fun. Emily Xie told me: “When it comes to generative art specifically, my analysis does involve one more layer, which considers technique. I often do find myself looking longer at generative works where it’s not quite clear to me what foundational or underlying techniques they are using.” The artist’s articulation of this conversation can be bold or muted, revealing strokes on a canvas the eye cannot see. Listening intently to the artist’s voice demonstrates the active dialogue between the viewer and artist that this essay advocates.
Understand the history
In addition to computer graphics industry pioneers like Loren Carpenter and Dan Sandin, the demoscene played a monumental role in the history of creative algorithms. Demosceners were highly skilled at creating real-time audiovisual graphics with extremely limited code space and no external assets. These coding wizards pioneered many techniques now commonplace today thanks to their ingenuity and code-space frugality, including SDFs and Raymarching, as previously mentioned. González Vivo explains, “There's a strong relationship between the shader world and the demoscene. A lot of old demosceners—like Inigo Quilez, Mr. Doob and Jaume Sanchez (Clicktorelease)—met through shaders. I have found them in my work career around places like Samsung, Apple, Adobe and Oculus. That bunch is very interesting because the mentality was very focused on visual and technical hacking in order to make cool, flashy demos.”
Knowing the history also means recognizing the art historical dialogue inspired by the algorithm or method. González Vivo elucidates, “It’s important to understand the history of those algorithms and then how they were reimagined along the way. I'm thinking about vector [flow] fields and the work of Jeremy Rotsztain in the late 2000s.” He compares the work to Hobbs’s Fidenza (2021), adding that “for collectors and artists, the interesting part is how the artists are communicating with each other through their work. That will help you detect the ‘Hello World’ version of certain techniques compared to something that feels mature. And it's mature artistically because it's based on this lineage.”

González Vivo highlights the criticality of observing the inner dialogue of these algorithms across time. On cue, Rotsztain himself credits Gustav Klimt in his description of Obsessions (Flickr Pets), pictured above from 2008. Yet the painterly images also recall the imposing gestural work of Joan Mitchell. Rotsztain and Hobbs’s conversation via flow fields is a reverberation of countless artistic dialogues throughout the ages, including Duchamp’s conversation with Cubism, Futurism and early experimental photography in his Nude Descending a Staircase, No. 2. Rotsztain's work with flow fields—and others’ in between—enriches the story of Fidenza. The fact that Fidenza is a generative system capable of producing one thousand unique outputs must also be taken into consideration. These deeper roots entrench work in art history, placing them in a centuries-long discussion.
Viewers, appreciators and artists should keep in mind what this new iteration is saying and showing. What is it contributing to this decades-long dialogue? What is the next sentence it adds to the story we’re so fascinated by?
4. Closing
Appreciating systems means participating in a dialogue with the artist on a level other than sensory perception. In systems, artistic expression extends beyond visuals, whispering with seductive visual outputs, yet when you get closer, you realize it’s not a whisper at all. It’s the rumblings of a lively conversation—with us, the past and their contemporaries. It’s our choice whether we participate or not.
Read Part I: Demystifying Generative Art
Read Part II: Demystifying Generative Aesthetics
Read Part IV: Demystifying Generative Autonomy
---
Peter Bauman (Monk Antony) is Le Random's Editor-in-Chief.